Successful use of real-time data transforms industrial operations and elevates data-driven decisions. But not so fast, success requires 3 activities.
Authored by: Justin Conroy, Vice President, Digital Product Portfolio - Radix.
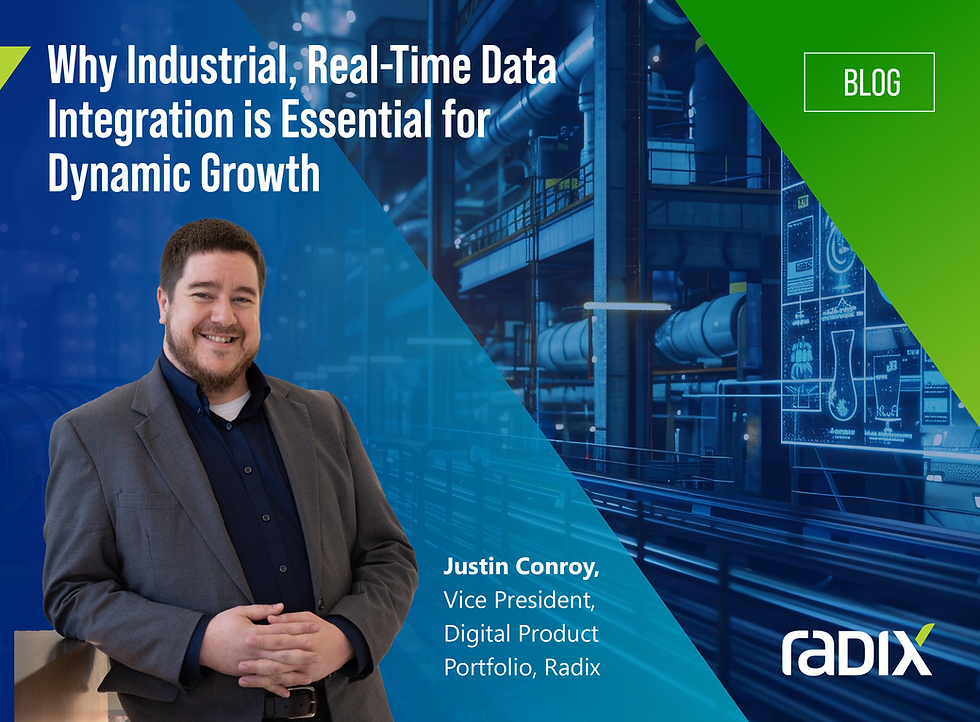
“You can’t manage what you don’t measure.” This sage comment resonates as true today as it did when I first read it in an old Harvard Business Review study, over a decade ago.
In today's data-driven world, real-time data has become a critical component in optimizing asset performance. By leveraging the latest technologies, enterprises can act on data as it is generated, leading to significant improvement in their operations.
Real-time data enables continuous monitoring of asset health, allowing enterprises to detect anomalies and potential issues before they escalate into major problems.
With the ability to access live data from sensors and other IoT devices, maintenance teams can track performance of machinery and infrastructure, ensuring they operate within optimal parameters.
As an example, a chemical processing plant can use real-time data to monitor flow rates, temperatures, and pressures of various processes, ensuring they operate at peak efficiency. This not only reduces energy consumption but also minimizes waste and improves product quality.
“Caveat emptor!” or as we translate from Latin, “let the buyer beware!” Achieving real-time data integration is a journey fraught with costs, delays, and challenges, unless you follow these next three steps.
Step 1: Choose Wisely
The first step in real-time data integration is selecting the right tools. When choosing tools, consider factors such as scalability, ease of integration, data security, ability to handle diverse data formats, and protocols.
Selection depends on the needs of an enterprise, existing infrastructure, and required outcomes. Essential tools include Extract, Transform, Load tools, data lakes, streaming analytics platforms, and data visualization tools.
Extract, Transform, Load tools, such as Azure Data Factory, AWS Glue, SAP Data Services, and others, are important for integrating data from multiple sources and transforming data into a usable format, before loading it into a centralized system.
For processing and analyzing data in real-time, event streaming platforms like Apache Kafka are useful. These platforms enable you to handle data as it streams from various sources.
Optimizing your data management strategy and approach, and leveraging these and other solutions, is made more effectively when partnering with experts who have a track record of robust data management and data optimization.
Step 2: Use What Works Efficiently
It sounds simple – but few do it. Integrating real-time data requires adherence to best practices to ensure data quality, consistency, and security and the enablement of data governance programs. An important practice is data normalization. By standardizing data from various sources, enterprises can ensure consistency and accuracy, making it easier to analyze and compare.
Establishing a unified data model is another best practice. This model defines how data is organized, stored, and accessed across the enterprise, ensuring that stakeholders have a collective understanding of data and its relationships.
Strong data quality measures are also important. Regular validation and cleansing of data helps prevent errors and inconsistencies, ensuring that data remains reliable.
In addition, data governance and security are vital. Developing a comprehensive data governance framework that defines roles, responsibilities, and policies for data management helps maintain data integrity. Ensuring data security measures are in place protects sensitive information from unauthorized access and breaches.
Step 3: Conquer Common Inhibitors
The path to real-time data integration has its share of challenges. One common obstacle is legacy system compatibility. Integrating data from outdated legacy systems is difficult. To address this, consider using middleware solutions or APIs to bridge the gap between legacy systems and modern data platforms. Gradual modernization of legacy systems can improve compatibility over time.
Data latency is another challenge that needs addressing. Minimizing latency is necessary for real-time integration. Optimizing network infrastructure, using data processing algorithms, and edge computing reduces the time taken for data to travel from source to destination.
Costs of real-time data integration are a concern, and such projects are expensive. To manage costs effectively, prioritize high-impact areas and start with pilot projects to demonstrate value. Leveraging cloud-based solutions reduces upfront investments.
What is at Stake?
By understanding diverse data sources, addressing data silos, and managing scalability issues, enterprises unlock the potential of their assets.
Real-time data integration offers the ability to continuously monitor asset health, predict maintenance needs, make informed decisions, and optimize resource utilization. These advantages translate into reduced downtime, cost savings, and enhanced operational efficiency - which are essential for any industry aiming to thrive in today's competitive landscape.
As industries evolve and the volume of data grows, those who embrace real-time data integration will be better positioned to achieve operational excellence and long-term growth.
To learn more, visit www.radixeng.com
Kommentare